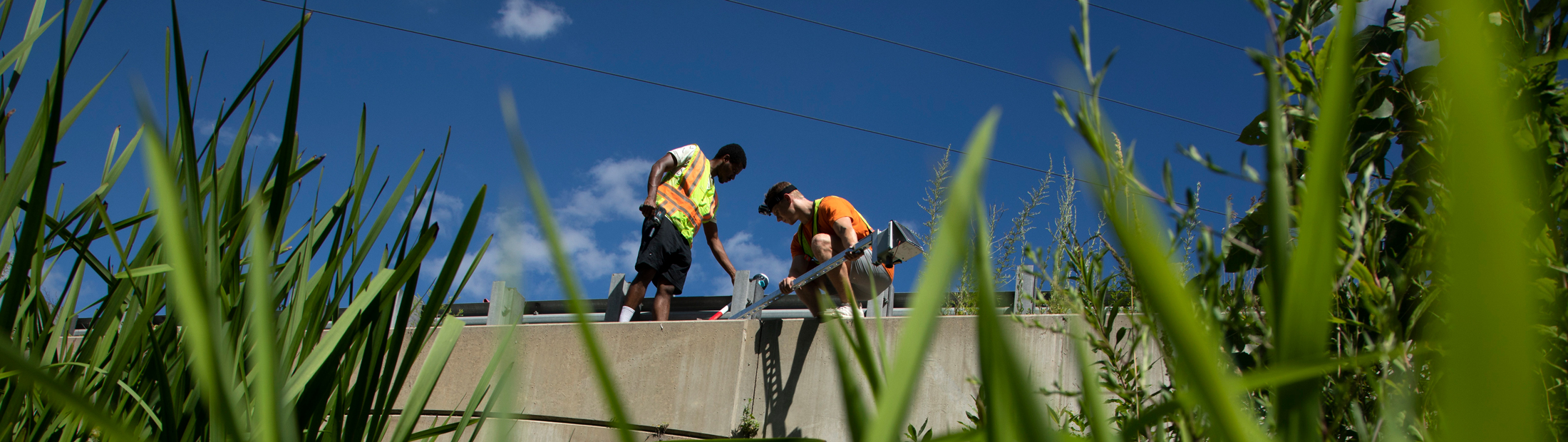
“Self-driving” water systems: A Q&A with Branko Kerkez
Taking a people-first approach to water management
Taking a people-first approach to water management
You don’t have to look very hard to find water problems.
More frequent and intense storms are causing increased flooding, particularly in rural and low-income communities, and can also lead to sewer overflows. Water treatment plants face new challenges from natural and human-generated contaminants. Infrastructure, such as dams that were built to control water, can have unintended consequences, disrupting ecosystems and changing migration patterns of fish.
Branko Kerkez, an Arthur F. Thurnau Associate Professor of civil and environmental engineering, and his students at the Digital Water Lab are tackling these water problems and more with inspiration from autonomous systems that originated in transportation, robotics or other fields. Like a self-driving car, water systems will one day “steer” themselves in real time based on changing conditions.
Guided by fundamental research questions, collaborations with experts in non-engineering fields and a focus on listening to and working with the people they aim to serve, Kerkez’s work exemplifies the Michigan Engineering people-first framework. In a Q&A, he shares how he takes a people-first approach to his work, and why it matters to him.
The Digital Water Lab at U-M focuses on making tech for water. A lot of our work is trying to untangle the relationship between water management and the kind of modern technologies that are already underpinning many other sectors of society. We imagine that similar tech to what’s in self-driving cars, robots and smart homes will one day play a critical role in managing some of our greatest water challenges.
We have a project in Detroit where there’s been a lot of flooding. We’re deploying sensors with the Sierra Club and Friends of the Rouge—two local community groups—measuring how resident-led backyard interventions might help reduce flooding. We’re also working with managers at the Great Lakes Water Authority on the real-time control of different water assets—basins and pumps and gates—and trying to figure out how to build tools for water operators to reduce sewer overflows and improve water treatment.
In another project on the Huron River, we’re trying to figure out how to enable entire smart river basins. We’ve been working with our collaborators at the Huron River Watershed Council—Ric Lawson and Andrea Paine—to figure out how to support the control of dams and reservoirs using sensors and data. The Huron River Watershed covers 900 square miles and tons of different rural and urban communities, who are all managing water in a vacuum in the sense that they’re making the best decisions they can, given what they know. Dam and reservoir operators are opening and closing gates based on whatever local needs they have, and all those flows can add up downstream in unintended ways. High or suddenly changing flows can cause ecological issues like flushing out the fish habitat in the springtime. We’re putting sensors out, gathering data about what’s happening and then building the tools for communities to make decisions about water flows. Across the country, dams are often viewed as environmental liabilities, but we believe that liabilities can become assets when coordinated across entire landscapes.
For a long time, we were doing the work that we thought was important and fun. We made progress on all these cool algorithms—essentially, smart city research that’s built on fundamental engineering. But right before the pandemic, I had a conversation with a few PhD students that were graduating. After validating the tech and showing its potential, what should we do next? Where is the potential to make the biggest impact?
The inclination is to take what you have and improve it. But what’s the value of pushing the performance of your control algorithm by 20% when few people are using the original one to begin with? There’s a massive chasm between what we make as researchers and what gets used in practice. Adoption hinges on many factors, and the technology itself may not even be the main hurdle to overcome. It’s humbling and uncomfortable when a whole room of engineering PhD students tells you that we probably need to understand people more than we need to understand the technology.
“I made this for you, you’re welcome,” is how I think of our traditional approach, which I think is kind of reflective of most of engineering. It’s much harder to ask before you build, “If I made this, would you want it?” There’s an inherent risk of rejection there, but not hedging against that—even just a little—risks us making something that does not provide value. Once we went down that path, we also started really nuancing what was useful versus usable. Sensors and data are inherently useful, most people can get on board with that. But can I take this powerhouse of a technology and actually package it in a way that someone can use day-to-day, and in the midst of all their other responsibilities? It turns out that’s a pretty hard problem to solve.
Every one of our projects is still rooted in a fundamental research question about something we’re trying to learn or algorithms we’re trying to put together, but now it’s always informed by what we’re learning on the ground. We start every research project now by spending time in the community and talking to residents and water managers before we formulate the research question. We always sort of did this implicitly, I think, but now it’s explicitly embraced as part of our approach. This helps us work on solutions that may actually be adopted because they’re solving a real problem for people.
Our lab is also a bit non-traditional in that a lot of the students have dual degrees in different technical disciplines like systems, environmental engineering, civil engineering, electrical engineering and computer science. Outside of the lab, things get really interesting because we have at the University of Michigan the Institute for Social Research, where we work with Associate Research Scientist Noah Webster to understand what the social barriers of adoption are for these tools we’re developing. For example, for dam operators, Noah will interview them and study their work patterns and social networks to try to see if or how new technology could make a difference in their day-to-day workflows.
Basically, in our lab we like to let the disciplines collide and see what happens. I realize that doesn’t sound overly pedagogical, but empirically it’s really led to cool discoveries and tools.
There’s an example that goes against how we typically do engineering research. The Great Lakes Water Authority operates a massive water distribution system and sewer system for the southeast Michigan area, serving millions of people. They make strategic decisions about how to build infrastructure and day-to-day operational decisions about which valve to open, who to send out to clean something, etc. We have research going back to 2017 that shows that if you change how you operate your pumps and gates and valves, you can save a lot of money—maybe billions of dollars—in infrastructure investments without even building something new. But that would require water operators to make the same exact decision that the algorithm suggests.
Some engineers call this a “human in the loop” problem. Oftentimes, when we talk about “the human,” it’s like they’re stopping us from doing what we think is optimal. But that terminology can be a little dismissive because it’s not drawing from the knowledge and experience of that person who’s making decisions, nor does it recognize that no matter how cool and shiny the thing is that you’re building, it’s not going to get adopted if people don’t trust it.
So we’re taking a social science approach to study how this group of water operators is organized and how they coordinate. We’re not trained as anthropologists or user experience researchers—far from it—but we’ve gotten really inspired by their methods. Some of our students are interviewing water operators to figure out what their day-to-day pain points and goals are. We even know which weather apps they use on their phones, which is a great way to learn where trust in technology sits. It’s often not with the most refined apps and engineering models, but rather just tools that help people do their job.
Without understanding the human component, it’s hard to make tools that are actually going to help users solve their problems. Users may not trust technology that behaves counter to intuition, but many algorithms seem to perform best when they don’t do what a human would do. How can we lift the hood on the self-driving water system to show people what’s inside, to let them form their own conclusions based on trust of the algorithm? Those kinds of questions allow us to prototype better, and have saved us a tremendous amount of time because we didn’t end up building something that’s useful but won’t be used.
Usually it’s word of mouth that trickles through an existing project. I can trace it back to literally my first week of this job. I was walking around trying to figure out where things are on campus, and I was introduced to the county water resource commissioner, Evan Pratt, at a coffee shop. He took me to the library the same day, where this group of residents was meeting to discuss water in their neighborhood. Collaborators like Evan help us see the bigger picture. He even helped us talk about our work in a more meaningful way. I’ll never forget him describing how real-time control and smart water helps him “squeeze” more performance out of his infrastructure. As if infrastructure is this malleable thing that you can squeeze to achieve some end goal. I guess when you connect it to the internet, it is. People like Evan help us talk about our work, and that’s half the battle sometimes.
I also spend a good amount of time going to local meetings, whether it’s a community college or a regional or statewide meeting of water professionals. Half the time I’m not presenting our technical research, I’m just showing pictures of work that we’re doing.
The University is really important because we have a tremendous amount of knowledge in house to benefit students, but there’s a lot to be learned outside of the building. Sometimes it’s stuff you don’t want to hear, like, “I don’t want the thing you’re making.” But, you know, you iterate.
One hard part is tying the work to fundamental research questions. A lot of times, the solutions that are needed on the ground aren’t the kind of stuff to write a research paper on. It’s hard because the students in our lab want to do both: they want to work in the community, but they also want to write PhD-level research papers. With enough looking and iteration, we often find a good overlap, though. It takes time. Until we figure out how to scale this approach or make it faster, in the meantime we’re just doing it because we think it’s a good thing to do.
Talk to people. Try to understand their pain points to see if there are slight adjustments you can make to ensure that the work that you’re doing has a positive impact. You don’t have to change your whole entire research program to fit it. Micro adjustments go a long way.
Community members and groups can be more than just test subjects or beta testers. They can be really meaningful collaborators in your research. The people that we work with give us feedback, and a lot of the ideas that we’ve worked on wouldn’t even exist if it weren’t for them. There are mutual benefits, but they may not be apparent from short interactions or initial conversations. It just requires spending some time—outside of the building.
Our core mission statement for the University says we’re here “to serve the people of Michigan and the world.” When I look at the day-to-day tasks that I do as a researcher, am I fulfilling that mission? Being a part of a big place like this means we can tackle global challenges, but there are cool things to work on in the state and our local communities as well.