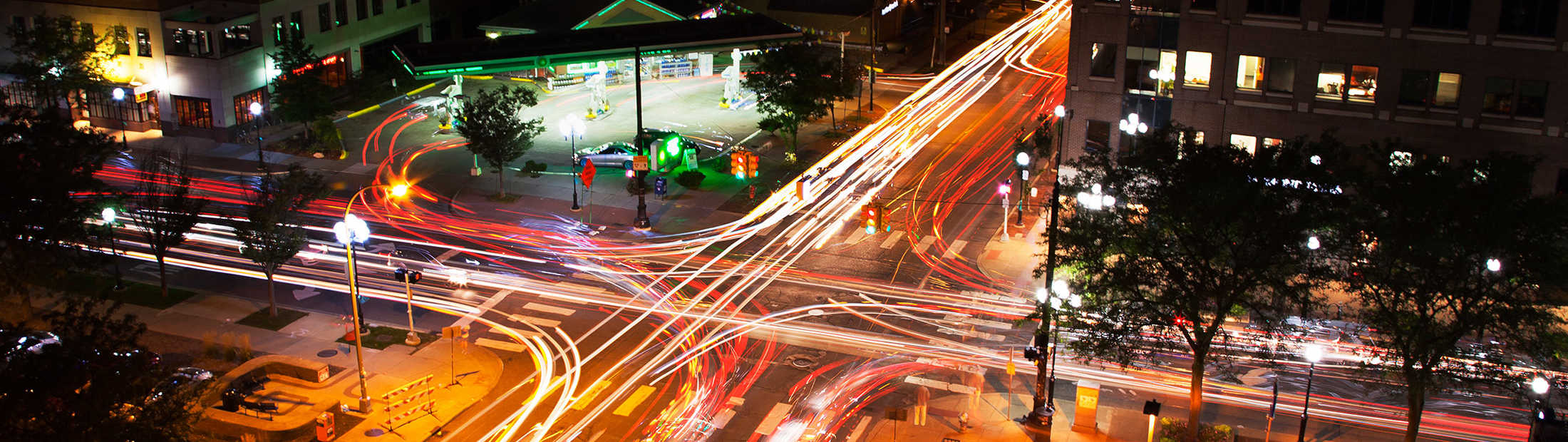
Transportation innovation
CEE Professors Henry Liu and Neda Masoud discuss their research on evolving transportation while keeping the public safe.
CEE Professors Henry Liu and Neda Masoud discuss their research on evolving transportation while keeping the public safe.
We’ve all been there – stuck in a long line of cars at a red light with no cross traffic in sight. The first electric traffic signal was installed in 1914. Over 100 years later, why does it seem like we still don’t know how to use them as well as we could? The short answer is lack of information. To gather data about traffic, engineers traditionally install sensors at intersections in the form of cameras, underground sensors called hoop detectors or radar. But those systems are costly to install and maintain.
Professor Henry Liu took a different approach to this problem. Rather than having a stationary sensor at an intersection, what if there were thousands of mobile sensors zipping along with traffic? Sounds ambitious, but in fact, these sensors already exist in the form of mobility services such as Uber and Lyft. “These services are already tracking this data for other reasons, to pay the driver for instance,” said Liu. “But that data can also be leveraged to study traffic performance and optimize traffic flow.”
Liu has developed software that optimizes vehicle trajectory data to help with traffic management. Working with Chinese mobility company DiDi Chuxing, he has already implemented this software in 1300 intersections in 20 cities in China. Liu believes that in the coming years, mobile sensors in the form of vehicles will make stationary sensors obsolete by providing more complete data to help government agencies improve traffic flow. Better traffic flow would also mean less congestion and less time spent on the road, and potentially reduced pollution from exhaust fumes.
Liu added, “I truly believe we are leading the world in this transition.”
While Professor Henry Liu’s plan is ideal for studying traffic in big cities with lots of shared mobility vehicles, other CEE faculty are examining traffic in different environments that present different challenges and opportunities. Gabor Orosz, CEE Associate Professor and Associate Professor of Mechanical Engineering, is working with Ford Motor Company to find a better way to report traffic data to drivers in real time. Right now, many people rely on apps or web services to predict traffic and help them find the fastest route to their destination. The main problem with these services is that they rely on aggregate data, which causes a delay in reporting.
“These services have to collect data from users’ devices, map that data, and relay it back to other users,” said Orosz. “By the time you see it on your phone, the traffic delay may be over. You see a red line on your map, but traffic is moving quickly in the real world.”
Orosz and his team piloted an alternative program on roads near Ann Arbor, Michigan. They leveraged sensors along the road that could communicate with connected vehicles, eliminating the need for aggregate data. Since the sensors connect directly to the vehicle, there is no delay in the information drivers are getting. Ten sensors were used in this pilot program, and the goal is to expand to hundreds of sensors to obtain better coverage. In developing this system, Orosz started by talking to drivers to understand their needs, how they drive and how they would want such a system to function.
“The technology is ultimately there to serve humans,” he said. “If we want it to help people, we need to get out there and talk to those people.”
But that doesn’t mean that this information is only useful for human-driven vehicles; it could also help automated vehicles improve their travel time and fuel economy. In fact, automated vehicles may make more efficient use of this information than human drivers. Automated vehicles responding to real-time traffic information would also help clear up traffic congestion, allowing all drivers to benefit from improved overall traffic flow.
The possibilities for connectivity and automation in vehicles and transportation infrastructure are tremendously exciting. But with this new technology comes the potential that these systems could be vulnerable to cyberattacks.
In the realm of autonomous and connected vehicles, a cyberattack could have far-reaching consequences. An autonomous and connected vehicle plans its trajectory based on the information collected through its sensors as well as information received from other connected vehicles and roadside sensors. If a vehicle cannot detect a cyberattack, not only would it impact the vehicle’s own trajectory but also the trajectory of other vehicles who are connected to it, either directly or through sensors embedded in infrastructure. The intent of an attack could range from a vehicle taking advantage of this connectivity to selfishly keep the traffic light green for its individual benefit, or an adversary trying to bring the traffic to a halt or even causing a vehicle to crash. Neda Masoud, CEE Assistant Professor, models connected vehicle systems to study the cybersecurity implications of this disruptive technology.
“When you have a connected system, if one sensor is hacked or has faulty information, that information will propagate through the network,” Masoud explained. “The goal with cybersecurity is to detect that anomaly or attack, identify the source and determine how to stop the spread of faulty information.”
Masoud uses sensor data collected in the Safety Pilot Model Deployment project conducted at the University of Michigan Transportation Research Institute. In this project, drivers volunteer to have wireless devices installed in their vehicles to test how well connected vehicle safety technologies and systems work in a realworld environment.
The data that Masoud receives comes from GPS devices, speedometers and accelerometers, collected at a frequency of ten messages per second. She introduces multiple types of attacks into this data to refine models that are best at identifying these attacks. These models use a combination of machine learning techniques (such as Convolutional Neural Networks and Support Vector Machines) and filters (such as Kalman Filters) to detect an attack, identify the faulty or attacked sensors and fuse the data from healthy sensors to generate accurate information that compensates for the missing data from the faulty or attacked sensors. In general, more sensors equal better cybersecurity – with redundant sensors, there are more copies of the same piece of data and less risk of generating faulty information. But this also means more data to process, more power consumption and more costly vehicles.
“It’s about balance, about finding the best combination of sensors to maximize safety and cybersecurity while minimizing power consumption in the vehicle,” said Masoud.
As autonomous and connected vehicles become more commonplace, consumers will start to consider cybersecurity features right alongside airbag performance and gas mileage. Future vehicles will need to incorporate cybersecurity models like Masoud’s in order to keep their passengers and other road users safe.
Marketing Communications Specialist
Department of Civil and Environmental Engineering